每天推薦一個 GitHub 優質開源項目和一篇精選英文科技或編程文章原文,歡迎關注開源日報。交流QQ群:202790710;電報群 https://t.me/OpeningSourceOrg
今日推薦開源項目:《命令行性能調優工具——Hyperfine》GitHub地址
推薦理由:這是一個命令行的性能調優工具(benchmarking tool),好用又酷到爆
演示:基準測試fd和find:
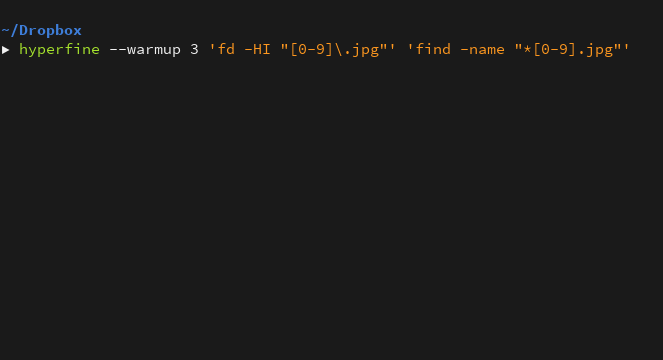
特性:
- 跨多個運行統計分析
- 支持任意的 shell 命令
- 不斷的反饋 benchmark 的進展和 current estimates
- 在 benchmarking 前可以進行 wormup run
- 在每一次測試前都可以設置緩存清理命令
使用:
可以簡單的調用hyperfine <command>…參數可以是任何 shell命令。 例如:
hyperfine 『sleep 0.3』
默認情況下, 它將執行至少10基準測試運行。也可以使用-m/–min-runs選擇:
hyperfine –min-runs 5 『sleep 0.2』 『sleep 3.2』
當然也可以進行I/O-heavy測試,這裡就不贅述了
今日推薦英文原文:《A look at open source image recognition technology》作者:Ben Cotton
原文鏈接:https://opensource.com/article/18/5/state-of-image-recognition
推薦理由:圖像識別也是很多同學關心的領域,那麼開源圖像識別技術的領域狀況如何呢?請看看《開源圖像識別技術一覽》
A look at open source image recognition technology
At the Supercomputing Conference in Denver last year, I discovered an interesting project as I walked the expo floor. A PhD student from Louisiana State University, Shayan Shams, had set up a large monitor displaying a webcam image. Overlaid on the image were colored boxes with labels. As I looked closer, I realized the labels identified objects on a table.
Of course, I had to play with it. As I moved each object on the table, its label followed. I moved some objects that were off-camera into the field of view, and the system identified them too.
When I asked Shams about the project, I was surprised to learn that he did not need to write any code to create it—the entire thing came together from open software and data. Shams used the Common Objects in Context (COCO) dataset for object recognition, reducing unnecessary classes to enable it to run on less powerful hardware. "Detecting some classes, such as airplane, car, bus, truck, and so on in the SC exhibition hall [was] not necessary," he explained. To do the actual detection, Shams used the You Only Look Once (YOLO) real-time object detection system.
The hardware was the only part of the setup that wasn't open. Shams used an NVIDIA Jetson TX2 module to run the detection. The TX2 is designed to act as an edge device for AI inference (as opposed to the more computationally intensive AI training). This $300 device allows live video analysis to happen away from central computing resources, which is key to applications like self-driving cars and other scenarios where network latency or bandwidth constraints require compute at the edge.
While this setup makes an interesting demonstration of the capabilities of live image recognition, Shams' efforts go far beyond simply identifying pens and coffee mugs. Working under LSU professor Seung-Jong Park, Shams is applying his research to the field of biomedical imaging. In one project, he applied deep learning to mammography: By analyzing mammogram images, medical professionals can reduce the number of unnecessary biopsies they perform. This not only lowers medical costs, but it saves patients stress.
Shams is also working on LSU's SmartCity project, which analyzes real-time data from traffic cameras in Baton Rouge to help detect criminal activities such as robbery and drunk driving. To address ethical considerations, Shams explained that all videos are discarded except those in which abnormal or criminal activity is detected. For these, the video for the specific vehicle or person is time-stamped, encrypted, and saved in a database. Any video the model flags as suspicious is reviewed by two on-site system administrators before being sent to the officials for further investigation.
In the meantime, if you're interested in experimenting with image-recognition technology, a Raspberry Pi with a webcam is sufficient hardware (although the recognition may not be immediate). The COCO dataset and the YOLO model are freely available. With autonomous vehicles and robots on the horizon, experience with live image recognition will be a valuable skill.
每天推薦一個 GitHub 優質開源項目和一篇精選英文科技或編程文章原文,歡迎關注開源日報。交流QQ群:202790710;電報群 https://t.me/OpeningSourceOrg