今日推荐开源项目:《测试驱动 learn-go-with-tests》
今日推荐英文原文:《What Is Machine Learning?》
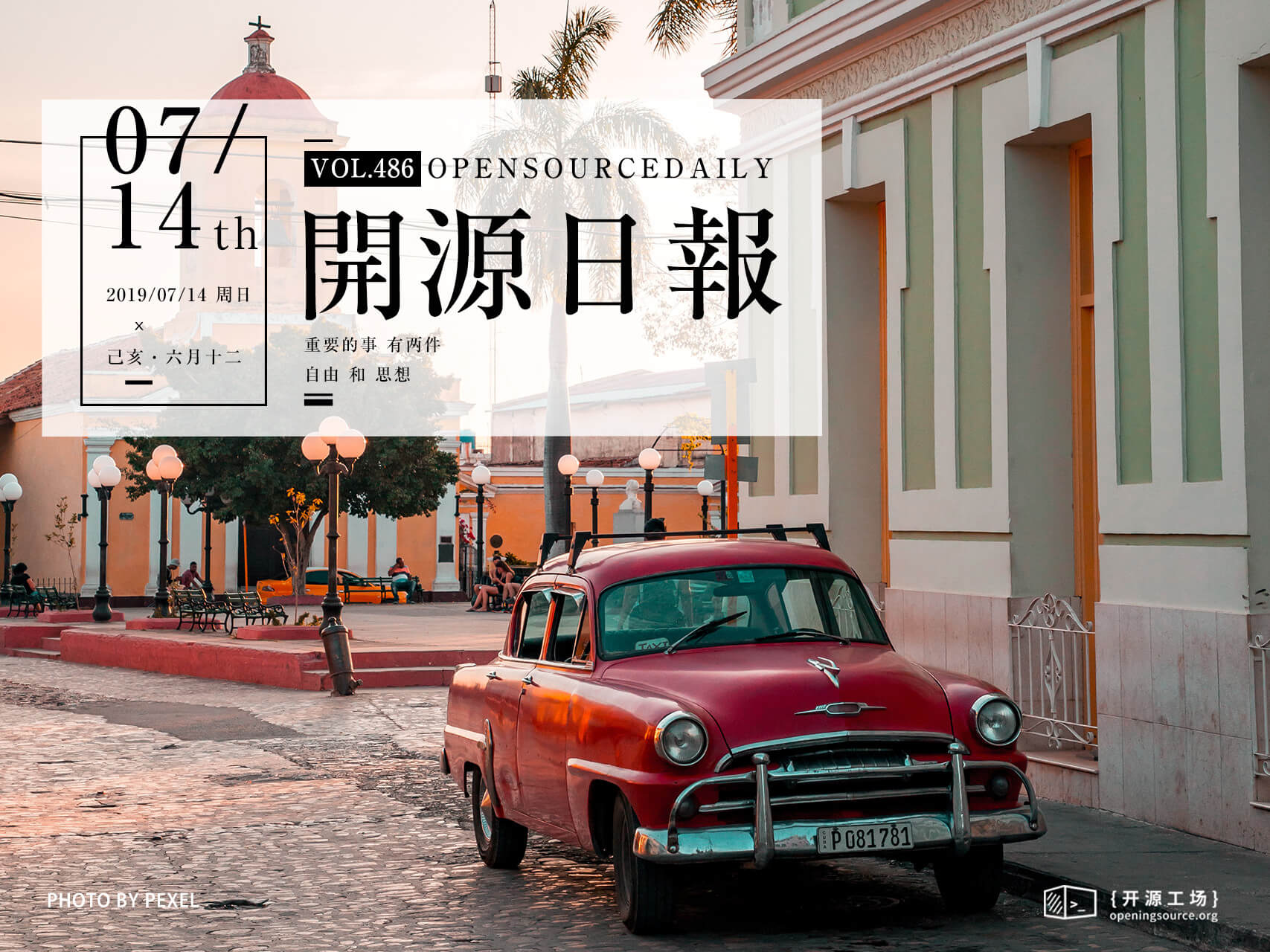
今日推荐开源项目:《测试驱动 learn-go-with-tests》传送门:GitHub链接
推荐理由:TDD 即测试驱动开发,即先编写测试用代码,然后让功能代码通过测试来不断前进的开发方法。这个项目就是使用 TDD 的方法来学习 Go 语言的教程,即不断的使用示例来帮助理解学习,而且对于熟悉 Go 内置的测试功能很有帮助。虽然总有一天测试代码会变的比功能代码都要多而且几乎不能真的拿来使用,但是在你不小心碰坏这个巨大积木塔的某一处时它们就会马上发现并指出来,在这层意义上多熟悉测试功能来写几个测试是没有坏处的。
今日推荐英文原文:《What Is Machine Learning?》作者:PCMag
原文链接:https://medium.com/pcmag-access/what-is-machine-learning-3c5f551f28c8
推荐理由:别忘了人工智能和机器学习并不是同一种东西
What Is Machine Learning?
Although it’s far from the original vision of artificial intelligence, machine learning has brought us much closer to the ultimate goal of creating thinking machines. Here’s how it works.By Ben Dickson
In December 2017, DeepMind, the research lab acquired by Google in 2014, introduced AlphaZero, an artificial intelligence program that could defeat world champions at several board games.
Interestingly, AlphaZero received zero instructions from humans on how to play the games (hence the name). Instead, it used machine learning, a branch of AI that develops its behavior through experience instead of explicit commands.
Within 24 hours, AlphaZero achieved superhuman performance in chess and defeated the previous world-champion chess program. Shortly after, AlphaZero’s machine-learning algorithm also mastered Shogi (Japanese chess) and the Chinese board game Go, and it defeated its predecessor, AlphaGo, 100 to zero.
Machine learning has become popular in recent years and is helping computers solve problems previously thought to be the exclusive domain of human intelligence. And even though it’s still a far shot from the original vision of artificial intelligence, machine learning has gotten us much closer to the ultimate goal of creating thinking machines.
What’s the Difference Between Artificial Intelligence and Machine Learning?
Traditional approaches to developing artificial intelligence involve meticulously coding all the rules and knowledge that define an AI agent’s behavior. When creating rule-based AI, developers must write instructions that specify how the AI should behave in response to every possible situation. This rule-based approach, also known as good old-fashioned AI (GOFAI) or symbolic AI, tries to mimic the human mind’s reasoning and knowledge representation functions.A perfect example of symbolic AI is Stockfish, a top-ranking, open-source chess engine more than 10 years in the making. Hundreds of programmers and chess players have contributed to Stockfish and helped develop its logic by coding its rules-for example, what the AI should do when the opponent moves its knight from B1 to C3.
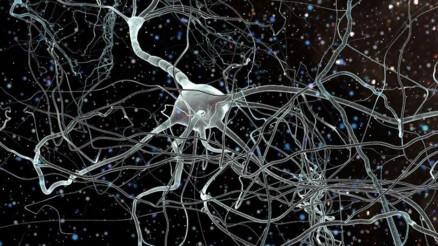
But rule-based AI often breaks when dealing with situations where the rules are too complex and implicit. Recognizing speech and objects in images, for instance, are advanced operations that can’t be expressed in logical rules.
As opposed to symbolic AI, machine-learning AI models are developed not by writing rules but by gathering examples. For instance, to create a machine learning-based chess engine, a developer creates a base algorithm and then “trains” it with data from thousands of previously played chess games. By analyzing the data, the AI finds common patterns that define winning strategies, which it can use to defeat real opponents.
The more games the AI reviews, the better it becomes at predicting winning moves during play. This is why machine learning is defined as a program whose performance improves with experience.
Machine learning is applicable to many real-world tasks, including image classification, voice recognition, content recommendation, fraud detection, and natural language processing.
Supervised and Unsupervised Learning
Depending on the problem they want to solve, developers prepare relevant data to build their machine-learning model. For instance, if they wanted to use machine learning to detect fraudulent bank transactions, developers would compile a list of existing transactions and label them with their outcome (fraudulent or valid). When they feed the data to the algorithm, it separates the fraudulent and valid transactions and finds the common characteristics within each of the two classes. The process of training models with annotated data is called “supervised learning” and is currently the dominant form of machine learning.Many online repositories of labeled data for different tasks already exist. Some popular examples are ImageNet, an open-source dataset of more than 14 million labeled images, and MNIST, a dataset of 60,000 labeled handwritten digits. Machine-learning developers also use platforms such as Amazon’s Mechanical Turk, an online, on-demand hiring hub for performing cognitive tasks such as labeling images and audio samples. And a growing sector of startups specialize in data annotation.
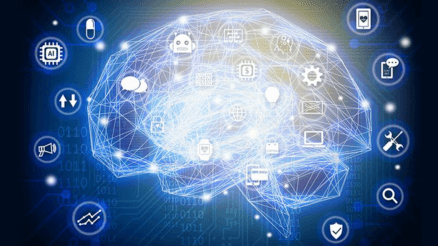
But not all problems require labeled data. Some machine-learning problems can be solved through “unsupervised learning,” where you provide the AI model with raw data and let it figure out for itself which patterns are relevant.
A common use of unsupervised learning is anomaly detection. For instance, a machine-learning algorithm can train on the raw network-traffic data of an internet-connected device-say, a smart fridge. After training, the AI establishes a baseline for the device and can flag outlier behavior. If the device becomes infected with malware and starts communicating with malicious servers, the machine-learning model will be able to detect it, because the network traffic is different from the normal behavior observed during training.
Reinforcement Learning
By now, you probably know that quality training data plays a huge role in the efficiency of machine learning models. But reinforcement learning is a specialized type of machine learning in which an AI develops its behavior without using previous data.Reinforcement-learning models start with a clean slate. They’re instructed only on their environment’s basic rules and the task at hand. Through trial and error, they learn to optimize their actions for their goals.
DeepMind’s AlphaZero is an interesting example of reinforcement learning. As opposed to other machine-learning models, which must see how humans play chess and learn from them, AlphaZero started only knowing the pieces’ moves and the game’s win conditions. After that, it played millions of matches against itself, starting with random actions and gradually developing behavioral patterns.
Reinforcement learning is a hot area of research. It’s the main technology used to develop AI models that can master complex games such as Dota 2 and StarCraft 2 and is also used to solve real-life problems such as managing data center resources and creating robotic hands that can handle objects with human-like dexterity.
Deep Learning
Deep learning is another popular subset of machine learning. It uses artificial neural networks, software constructions that are roughly inspired by the biological structure of the human brain.Neural networks excel at processing unstructured data such as images, video, audio, and long excerpts of text such as articles and research papers. Before deep learning, machine-learning experts had to put a lot of effort into extracting features from images and videos and would run their algorithms on top of that. Neural networks automatically detect those features without requiring much effort from human engineers.
Deep learning is behind many modern AI technologies such as driverless cars, advanced translation systems, and the facial-recognition tech in your iPhone X.
The Limits of Machine Learning
People often confuse machine learning with human-level artificial intelligence, and the marketing departments of some companies intentionally use the terms interchangeably. But while machine learning has taken great strides toward solving complex problems, it is still very far from creating the thinking machines envisioned by the pioneers of AI.In addition to learning from experience, true intelligence requires reasoning, common sense, and abstract thinking-areas in which machine learning models perform very poorly.
For instance, while machine learning is good at complicated pattern-recognition tasks such as predicting breast cancer five years in advance, it struggles with simpler logic and reasoning tasks such as solving high-school math problems.
Machine learning’s lack of reasoning power makes it bad at generalizing its knowledge. For instance, a machine-learning agent that can play Super Mario 3 like a pro won’t dominate another platform game, such as Mega Man, or even another version of Super Mario. It would need to be trained from scratch.
Without the power to extract conceptual knowledge from experience, machine-learning models require tons of training data to perform. Unfortunately, many domains lack sufficient training data or don’t have the funds to acquire more. Deep learning, which is now the prevalent form of machine learning, also suffers from an explainability problem: Neural networks work in complicated ways, and even their creators struggle to follow their decision-making processes. This makes it difficult to use the power of neural networks in settings where there’s a legal requirement to explain AI decisions.
Fortunately, efforts are being made to overcome machine learning’s limits. One notable example is a widespread initiative by DARPA, the Department of Defense’s research arm, to create explainable AI models.
Other projects aim to reduce machine learning’s over-reliance on annotated data and make the technology accessible to domains with limited training data. Researchers at IBM and MIT recently made inroads in the field by combining symbolic AI with neural networks. Hybrid AI models require less data for training and can provide step-by-step explanations of their decisions.
Whether the evolution of machine learning will eventually help us reach the ever-elusive goal of creating human-level AI remains to be seen. But what we know for sure is that thanks to advances in machine learning, the devices sitting on our desks and resting in our pockets are getting smarter every day.
下载开源日报APP:https://openingsource.org/2579/
加入我们:https://openingsource.org/about/join/
关注我们:https://openingsource.org/about/love/