今日推荐开源项目:《其他 CSS 能做到吗 css-only-chat》
今日推荐英文原文:《AI & Ethics: Are We Making It More Difficult On Ourselves?》
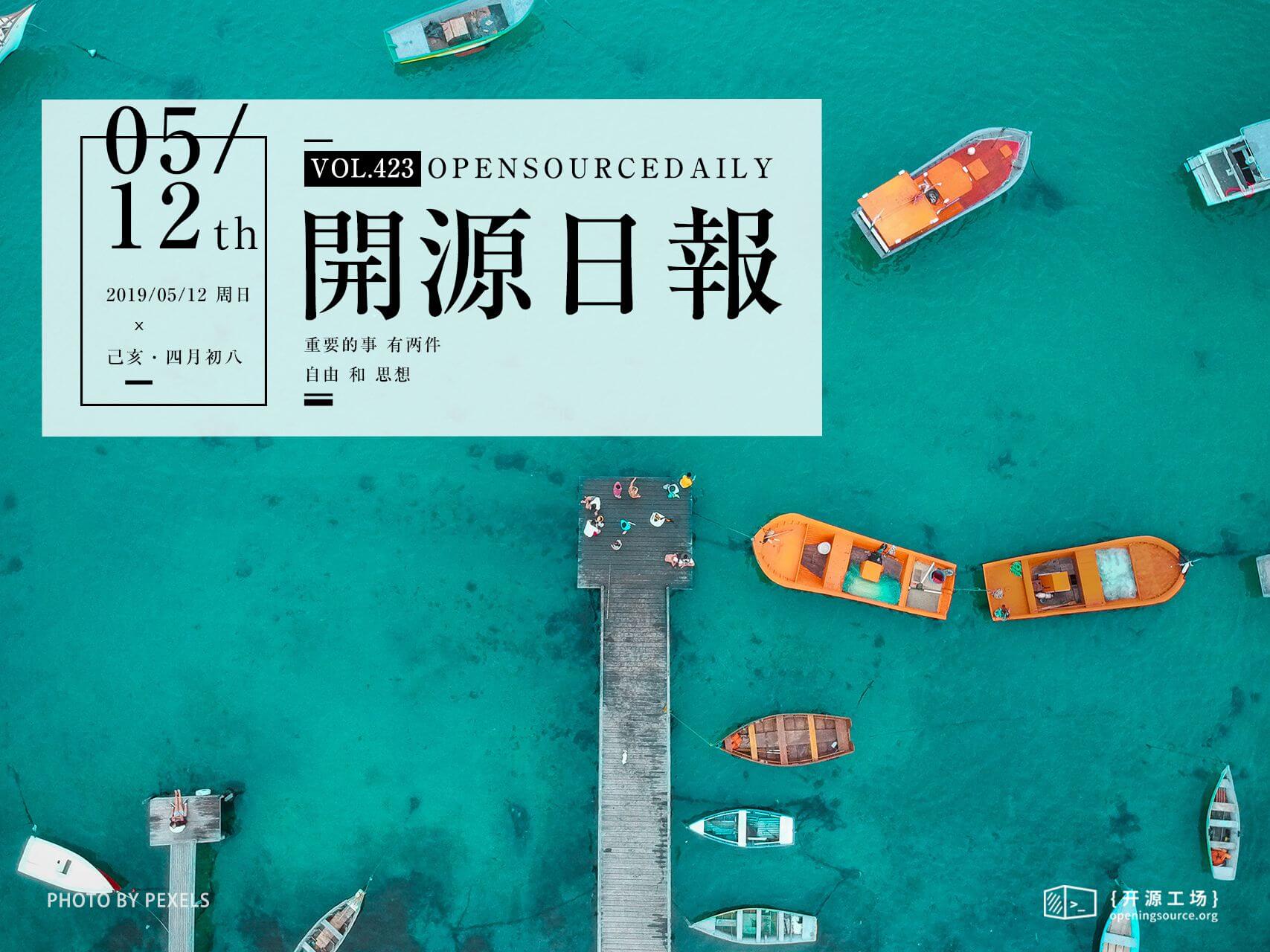
今日推荐开源项目:《其他 CSS 能做到吗 css-only-chat》传送门:GitHub链接
推荐理由:CSS 的能力是有极限的。我从短暂的人生当中学到一件事……越是玩弄代码,就越会发现 CSS 的能力是有极限的……
异议あり!CSS 的话,就算是即时聊天也做给你看!
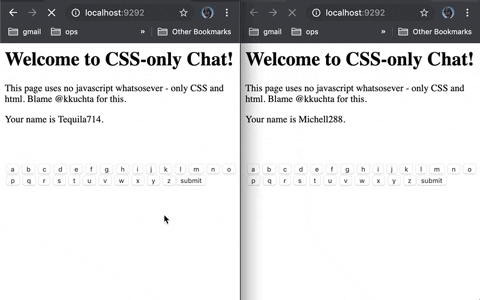
今日推荐英文原文:《AI & Ethics: Are We Making It More Difficult On Ourselves?》作者:Patrick McClory
原文链接:https://medium.com/@pmdev/ai-ethics-are-we-making-it-more-difficult-on-ourselves-2783e48c95d2
推荐理由:随着 AI 的不断发展,道德问题也变得越来越重要,兴许在以后 AI 也会拥有类似机器人三原则一样不可逾越的行为底线。
AI & Ethics: Are We Making It More Difficult On Ourselves?
Not too long ago we discussed the AI Apocalypse as it pertained to the Facebook #TenYearChallenge. Is Facebook evil? Are we evil for helping usher in our own demise? As we put it: not quite. However, AI & ethics seem inexorably linked and for good reason. This is part of an ongoing series on the question of AI and ethics. And there’s no better place to start than with science fiction, of course.The question of what artificial intelligence could be capable of has captured our imaginations for a long while. The truth is, the idea may stretch as far back, at least in concept, to Ancient Greece.
To get philosophical, the idea of what humankind’s creations could be capable of is not new. Neither is the notion of how we would contend with this. However, at least in a modern sense, Isaac Asimov was instrumental in thrusting the question into the public debate. At least as it pertains to artificial intelligence. Namely, robots. What could robots do? And how could we stop them?
Thankfully, Asimov had a solution. The Three Laws of Robotics were first introduced in the 1942 short story “Runaround.” Asimov provides a set of guidelines which were key components of all robot programming:
- First Law: A robot may not injure a human being or, through inaction, allow a human being to come to harm.
- Second Law: A robot must obey the orders given it by human beings except where such orders would conflict with the First Law.
- Third Law: A robot must protect its own existence as long as such protection does not conflict with the First or Second Laws.
Is that Enough?
The concept of AI & ethics is nothing new. Nor should it be. We believe strongly that anyone who occupies this space should be thinking, evaluating, and considering the ethical implications of AI. Today, as well as tomorrow.The notion of robots turnings on their creators makes for great science fiction. However, we aren’t quite there yet. And it’s a good thing, according to Scientific American which doesn’t think Asimov’s laws would even work:
While these laws sound plausible, numerous arguments have demonstrated why they are inadequate. Asimov’s own stories are arguably a deconstruction of the laws, showing how they repeatedly fail in different situations. Most attempts to draft new guidelines follow a similar principle to create safe, compliant and robust robots. ——By Christoph Salge, The Conversation US on July 11, 2017Our modern day concerns with regard to AI & ethics are typically less about robots taking over the world and more about securing data from theft, preventing algorithmic biases, and the responsible way in which we approach AI, data, and more.
True, it’s slightly less grandiose than worrying about how to keep us from building Skynet; but nonetheless there are important concerns regarding AI & ethics which warrant attention and scrutiny.
Recent articles have raised these questions yet again. Most recently 4 Ways AI Education and Ethics Will Disrupt Society in 2019 and perhaps more pointedly: Is It Possible For AI To Be Ethical?
Well, is it possible?
Yes. And No. But Mostly Yes. Maybe.
We’re generating a lot of data these days. A lot. And there are a lot of concerns of what we’re doing with that data. Understandably and smartly so. However, there are also concerns that we’re perhaps doing more harm than good. Or at least, from an endpoint perspective, we’re making things more difficult for ourselves.The General Data Protection Regulation (GDPR) which was implemented in the EU last year is one such example of something that is making things more difficult for us.
From certain perspectives, it’s hard to argue with the notions set forth in GDPR. Namely that organizations have an ethical responsibility to handle your data properly, not share it, and keep it protected. All good things in theory.
However, for better or for worse, it’s also a wall. And walls can undoubtedly keep bad things from getting in. However, it can also keep good things from getting out.
What GDPR succeeds in doing, partly by design and partly unintentionally, is to cordon off data from the outside world. Is that a good thing? Well, not always.
Contrast these ideas with widespread accusation and belief there are biased algorithms everywhere in Silicon Valley. That certain groups benefit from what should be (at least in certain minds) unbiased equations.
Now, consider how these algorithms are created. Or more importantly, where they are created.
The Walled Off Data Problem
In the past, we were accustomed to obtaining data from a single source. Or at least, very few sources. And by “data” we mean thousands upon thousands of bits of information that all put together creates a coherent, workable model of algorithmic goodness.The problem which is perhaps unintentionally created by restrictive data protection laws is that it makes data harder to come by legally. Because of concerns regarding AI & ethics, we’re walling off data like never before. Keeping it restricted.
Now that may not sound like a bad thing if your mind conjures up images of a telemarketing firm looking to create a model so they knew who to call and bother at dinnertime. It may be a bad thing if you’re a University Medical Research Department building a model to predict, diagnose, or even cure disease.
We’ve spoken at length in the past about “the silo problem” as it relates to development and deployment. Specialized teams are able to exhibit hyper-focused attention to one specific aspect of the problem. However, it doesn’t necessarily yield the best results or the best end product.
The same can be said of approaching data in a silo. To tackle the world’s problems, or even attempt to do so, we need access to a lot of data. And as growing restrictions further cordon off that data, we run the risk of biasing our own data pool.
To be clear: when we talk about being able to gather data in one place: we mean a wide array of data which is accessible from a single source; but not a wide array of data that originates from a single source.
Let’s Bake Some Bread
For example, it’s great to be able to go to a supermarket where we can purchase, bread, milk, meat, and vegetables all in one place. The supermarket is a great source of a lot of different types of products (data). If we wanted to build an algorithm to track or predict what groceries people purchase, a supermarket would be a good place to start.Why? Because we know that the shoppers there are going to purchase a wide variety of items, across different types and variants. We’ll be able to view a veritable ton of data to build our model.
Now, let’s suppose that supermarkets didn’t exist. Indeed, it may be seen as “safer” or “better” to get your milk from a milkman, your produce from a vegetable market, or your bread from a baker specifically. However, it’s far less convenient and far more restrictive.
If you are purchasing your bread from a single source: you are beholden to that single source and all the characteristics of that source. How then, are we do build a model to track grocery purchases when we only have easy access to the baker’s data?
This is how we wind up unintentionally biasing our own algorithms.
Open Boarders Data
This is not to say there is not culturally significant social biases that can be built into algorithms or data practices. They absolutely can be. However, it is becoming increasingly difficult to build culturally significant and culture-spanning models because of the increasing difficulty in legally obtaining data across certain roadblocks.As a result, a model built in Silicon Valley might reflect the demography of Silicon Valley. A model built in India might reflect the demography of India, and so on. And one of the issues we are faced with is this one-size-fits-all approach is that it becomes difficult to meaningfully create a model from a set of data which may not reflect all users, all components, or even reach a realistic, ideal, or meaningful outcome if the data has been previously biased in one way or another.
Again, not a bad thing if we’re stopping telemarketing in most people’s eyes. It can be a bad thing if we’re using concerns of AI & ethics to cut off our own nose to spite our face.
The future of data collection and analysis is likely to look more like this: collect locally, repeat globally. It’s a longer and more involved process to be sure. However, the greater the push for enhanced data protection, the more restrictive access will be come.
So What Do We Do?
To a large degree, the conversation over AI & ethics is just getting started. And that’s a good thing. Because as we said earlier, we believe there’s an inherent responsibility for those who operate in this space to continue to ask these questions. Namely, are we behaving ethically? Are we contributing meaningful thought as well as action to the public space and public debate surrounding these questions. As the technologies evolve, these questions need to continue to be asked.To a degree, we believe that personal (and corporate responsibility) have to come into play. Government regulation can and will assist in pointing out the correct path. However, it will come with its own drawbacks and downsides as mentioned above.
There are good reasons for wanting regulation such as GDPR and the tightening regulations in the USA as well. However, there are unintentional downsides such as those outlined above. It also makes it difficult for newcomers to the space to get started. This relegates operations to a select few who have the means, resources, an connections to move in this space.
To a degree, the ethical treatment of AI may ultimately rest with those who control it. We may be a long way off from having to realistically worry about a robot uprising. Thankfully. That doesn’t mean there aren’t concerns with regard to bad actors in this space.
We have a responsibility to use AI responsibly. That doesn’t mean there won’t be mistakes, missteps, and mishaps along the way. It would be foolish to think otherwise. However, the question of AI and ethics is also a fundamentally human one. As human as the human beings who write the code which implements Asimov’s Three Laws of Robotics.
What happens when a bad actor “forgets” or omits this code? What happens when those charged with safeguarding data seek to misuse it? Not to wax too philosophical, but the question surrounding how ethical AI can be will, for the time being, rest ultimately within the confines of the ethical possibilities of human behavior.
We, of course, have free will. Unlike our robot underlings. For now.
下载开源日报APP:https://openingsource.org/2579/
加入我们:https://openingsource.org/about/join/
关注我们:https://openingsource.org/about/love/