每天推薦一個 GitHub 優質開源項目和一篇精選英文科技或編程文章原文,歡迎關注開源日報。交流QQ群:202790710;微博:https://weibo.com/openingsource;電報群 https://t.me/OpeningSourceOrg
今日推薦開源項目:《很大的幻燈片 big》GitHub鏈接
推薦理由:這個項目可以讓你用簡單的方式做一個很大的幻燈片,而且很簡單,你只需要使用一個瀏覽器即可打開它。你可以加入大的文字,大的圖片,大的鏈接,你甚至可以加大的代碼進去,適合那些在幻燈片上追求簡單或者想要偷懶的朋友。
大概這麼大:
今日推薦英文原文:《What exactly can you do with Python? Here are Python』s 3 main applications.》作者:YK Sugi
原文鏈接:https://medium.freecodecamp.org/what-can-you-do-with-python-the-3-main-applications-518db9a68a78
推薦理由:顧名思義,這篇文章講的是 Python3 到底能拿來幹啥的。作者在文中列出了腳本,Web 開發和數據科學三點,如果你有這些需求,可以試試在 Python 上加些技能點,興許它會讓你事半功倍。
What exactly can you do with Python? Here are Python』s 3 main applications.
If you』re thinking of learning Python — or if you recently started learning it — you may be asking yourself:
「What exactly can I use Python for?」
Well that』s a tricky question to answer, because there are so many applications for Python.
But over time, I have observed that there are 3 main popular applications for Python:
- Web Development
- Data Science — including machine learning, data analysis, and data visualization
- Scripting
Let』s talk about each of them in turn.
Web Development
Web frameworks that are based on Python like Django and Flask have recently become very popular for web development.
These web frameworks help you create server-side code (backend code) in Python. That』s the code that runs on your server, as opposed to on users』 devices and browsers (front-end code). If you』re not familiar with the difference between backend code and front-end code, please see my footnote below.
But wait, why do I need a web framework?
That』s because a web framework makes it easier to build common backend logic. This includes mapping different URLs to chunks of Python code, dealing with databases, and generating HTML files users see on their browsers.
Which Python web framework should I use?
Django and Flask are two of the most popular Python web frameworks. I』d recommend using one of them if you』re just getting started.
What』s the difference between Django and Flask?
There』s an excellent article about this topic by Gareth Dwyer, so let me quote it here:
<begin quote>
Main contrasts:
- Flask provides simplicity, flexibility and fine-grained control. It is unopinionated (it lets you decide how you want to implement things).
- Django provides an all-inclusive experience: you get an admin panel, database interfaces, an ORM [object-relational mapping], and directory structure for your apps and projects out of the box.
You should probably choose:
- Flask, if you』re focused on the experience and learning opportunities, or if you want more control about which components to use (such as what databases you want to use and how you want to interact with them).
- Django, if you』re focused on the final product. Especially if you』re working on a straight-forward application such as a news site, an e-store, or blog, and you want there to always be a single, obvious way of doing things.
</end quote>
In other words, If you』re a beginner, Flask is probably a better choice because it has fewer components to deal with. Also, Flask is a better choice if you want more customization.
Also, according to my data engineer friend Jonathan T Ho, Flask is more suited for creating these things called REST API』s than Django due to its flexibility.
On the other hand, if you』re looking to build something straight-forward, Django will probably let you get there faster.
Okay, let』s go to the next topic!
Data Science — including machine learning, data analysis, and data visualization
First of all, let』s review what machine learning is.
I think the best way to explain what machine learning is would be to give you a simple example.
Let』s say you want to develop a program that automatically detects what』s in a picture.
So, given this picture below (Picture 1), you want your program to recognize that it』s a dog.
Given this other one below (Picture 2), you want your program to recognize that it』s a table.
You might say, well, I can just write some code to do that. For example, maybe if there are a lot of light brown pixels in the picture, then we can say that it』s a dog.
Or maybe, you can figure out how to detect edges in a picture. Then, you might say, if there are many straight edges, then it』s a table.
However, this kind of approach gets tricky pretty quickly. What if there』s a white dog in the picture with no brown hair? What if the picture shows only the round parts of the table?
This is where machine learning comes in.
Machine learning typically implements an algorithm that automatically detects a pattern in the given input.
You can give, say, 1,000 pictures of a dog and 1,000 pictures of a table to a machine learning algorithm. Then, it will learn the difference between a dog and a table. When you give it a new picture of either a dog or a table, it will be able to recognize which one it is.
I think this is somewhat similar to how a baby learns new things. How does a baby learn that one thing looks like a dog and another a table? Probably from a bunch of examples.
You probably don』t explicitly tell a baby, 「If something is furry and has light brown hair, then it』s probably a dog.」
You would probably just say, 「That』s a dog. This is also a dog. And this one is a table. That one is also a table.」
Machine learning algorithms work much the same way.
You can apply the same idea to:
- recommendation systems (think YouTube, Amazon, and Netflix)
- face recognition
- voice recognition
among other applications.
Popular machine learning algorithms you might have heard about include:
- Neural networks
- Deep learning
- Support vector machines
- Random forest
You can use any of the above algorithms to solve the picture-labeling problem I explained earlier.
Python for machine learning
There are popular machine learning libraries and frameworks for Python.
Two of the most popular ones are scikit-learn and TensorFlow.
- scikit-learn comes with some of the more popular machine learning algorithms built-in. I mentioned some of them above.
- TensorFlow is more of a low-level library that allows you to build custom machine learning algorithms.
If you』re just getting started with a machine learning project, I would recommend that you first start with scikit-learn. If you start running into efficiency issues, then I would start looking into TensorFlow.
How should I learn machine learning?
To learn machine learning fundamentals, I would recommend either Stanford』s or Caltech』s machine learning course.
Please note that you need basic knowledge of calculus and linear algebra to understand some of the materials in those courses.
Then, I would practice what you』ve learned from one of those courses with Kaggle. It』s a website where people compete to build the best machine learning algorithm for a given problem. They have nice tutorials for beginners, too.
What about data analysis and data visualization?
To help you understand what these might look like, let me give you a simple example here.
Let』s say you』re working for a company that sells some products online.
Then, as a data analyst, you might draw a bar graph like this.
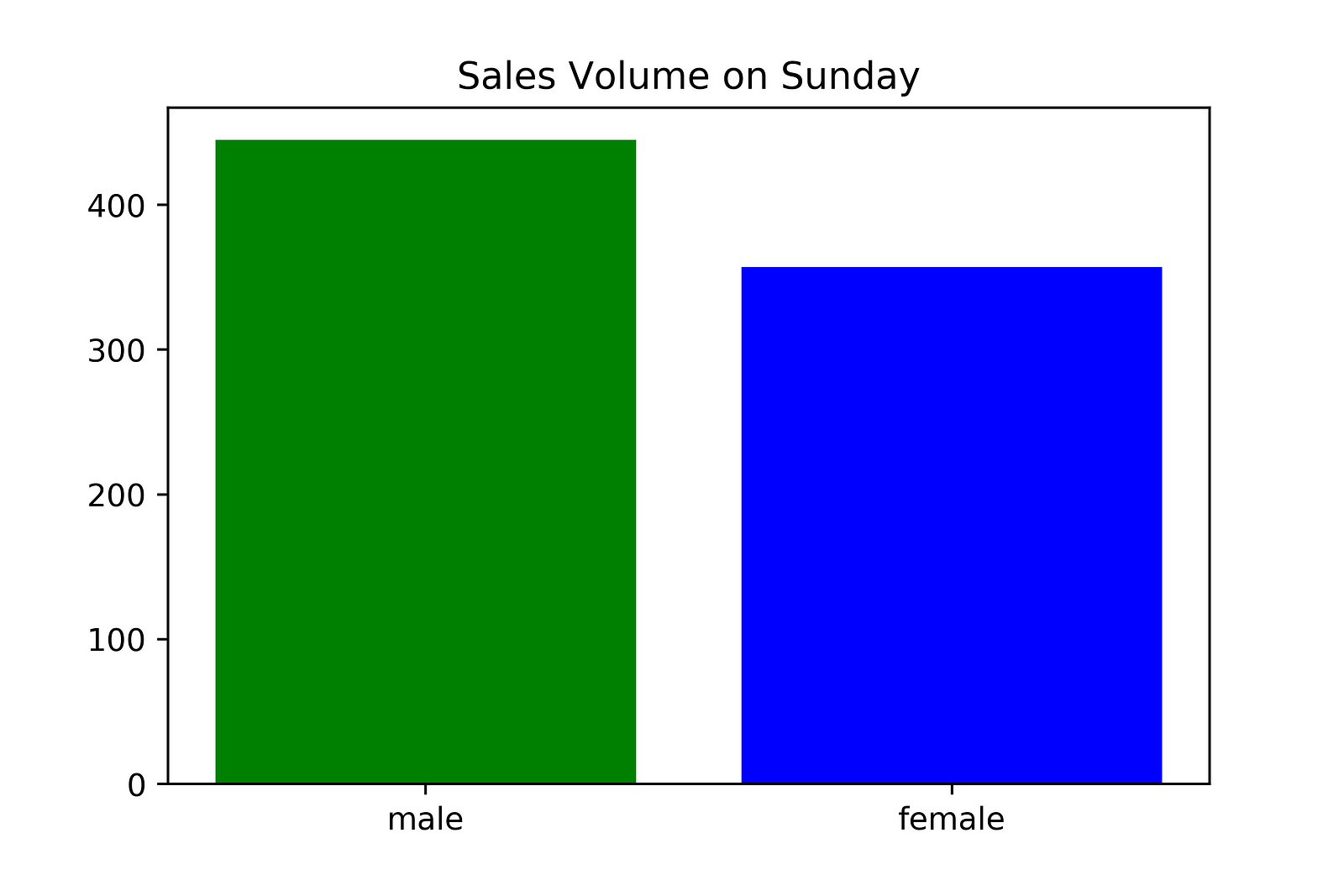
From this graph, we can tell that men bought over 400 units of this product and women bought about 350 units of this product this particular Sunday.
As a data analyst, you might come up with a few possible explanations for this difference.
One obvious possible explanation is that this product is more popular with men than with women. Another possible explanation might be that the sample size is too small and this difference was caused just by chance. And yet another possible explanation might be that men tend to buy this product more only on Sunday for some reason.
To understand which of these explanations is correct, you might draw another graph like this one.
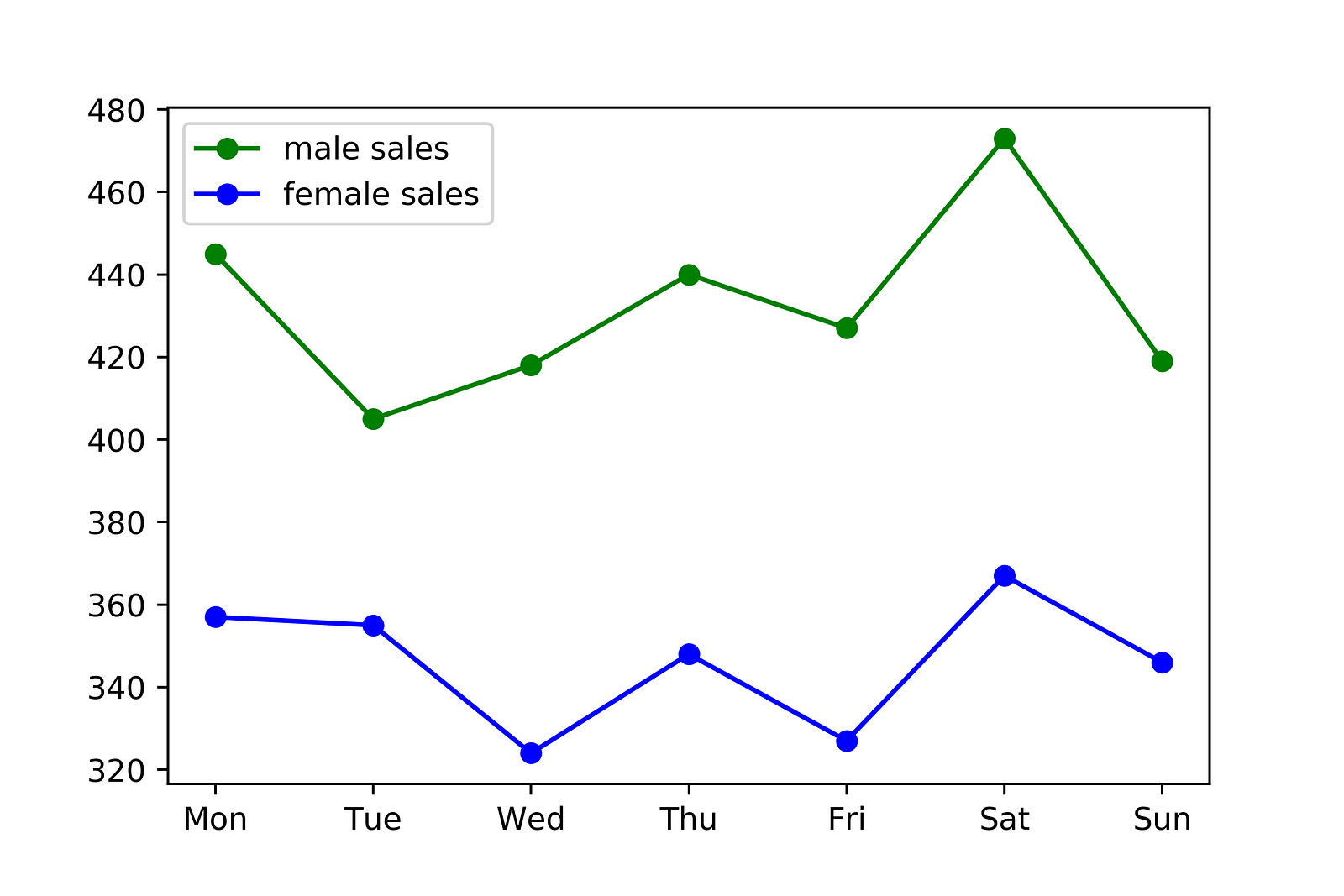
Instead of showing the data for Sunday only, we』re looking at the data for a full week. As you can see, from this graph, we can see that this difference is pretty consistent over different days.
From this little analysis, you might conclude that the most convincing explanation for this difference is that this product is simply more popular with men than with women.
On the other hand, what if you see a graph like this one instead?
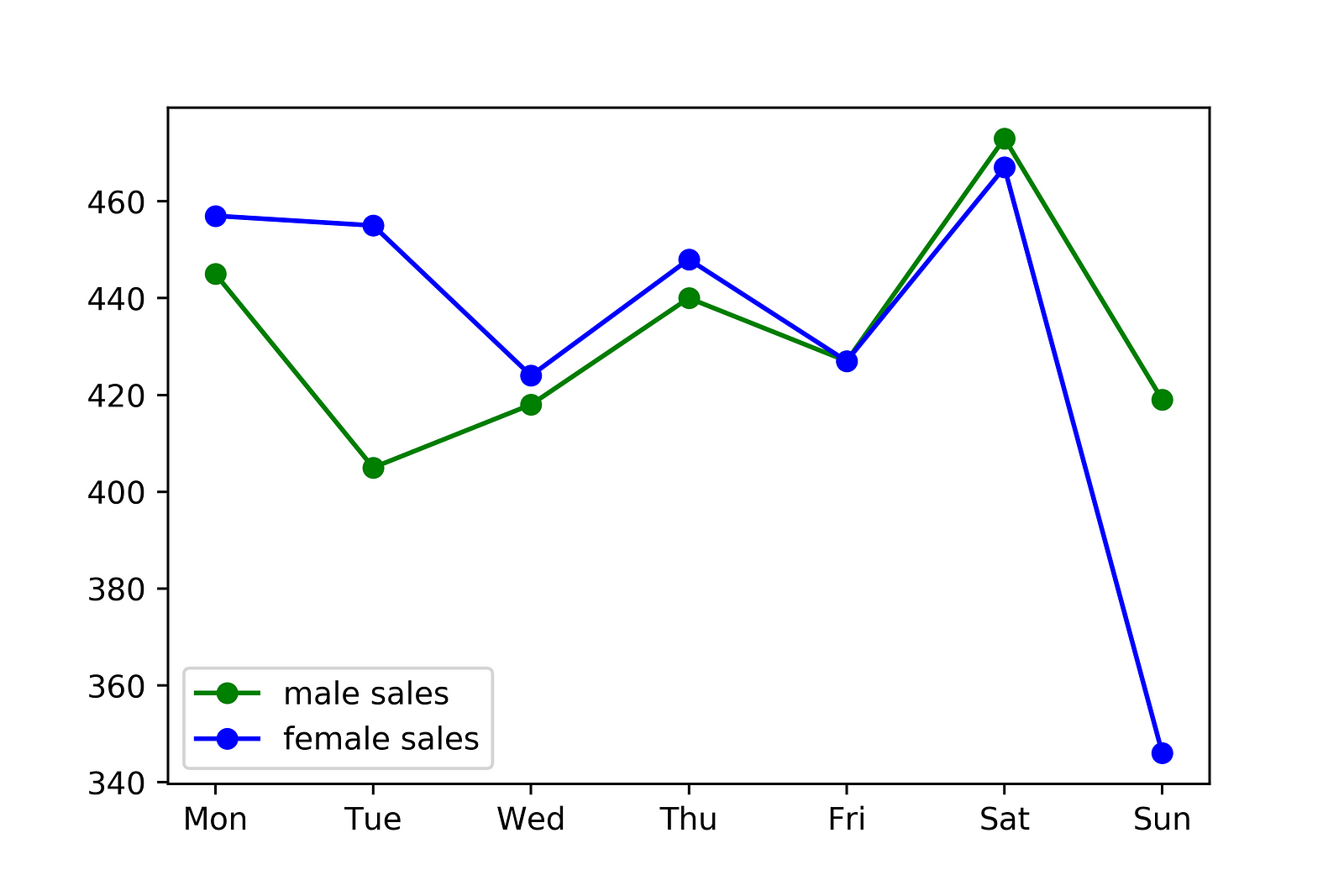
Then, what explains the difference on Sunday?
You might say, perhaps men tend to buy more of this product only on Sunday for some reason. Or, perhaps it was just a coincidence that men bought more of it on Sunday.
So, this is a simplified example of what data analysis might look like in the real world.
The data analysis work I did when I was working at Google and Microsoft was very similar to this example — only more complex. I actually used Python at Google for this kind of analysis, while I used JavaScript at Microsoft.
I used SQL at both of those companies to pull data from our databases. Then, I would use either Python and Matplotlib (at Google) or JavaScript and D3.js (at Microsoft) to visualize and analyze this data.
Data analysis / visualization with Python
One of the most popular libraries for data visualization is Matplotlib.
It』s a good library to get started with because:
- It』s easy to get started with
- Some other libraries such as seaborn is based on it. So, learning Matplotlib will help you learn these other libraries later on.
How should I learn data analysis / visualization with Python?
You should first learn the fundamentals of data analysis and visualization. When I looked for good resources for this online, I couldn』t find any. So, I ended up making a YouTube video on this topic:
I also ended up making a full course on this topic on Pluralsight, which you can take for free by signing up to their 10-day free trial.
I』d recommend both of them.
After learning the fundamentals of data analysis and visualization, learning fundamentals of statistics from websites like Coursera and Khan Academy will be helpful, as well.
Scripting
What is scripting?
Scripting usually refers to writing small programs that are designed to automate simple tasks.
So, let me give you an example from my personal experience here.
I used to work at a small startup in Japan where we had an email support system. It was a system for us to respond to questions customers sent us via email.
When I was working there, I had the task of counting the numbers of emails containing certain keywords so we could analyze the emails we received.
We could have done it manually, but instead, I wrote a simple program / simple script to automate this task.
Actually, we used Ruby for this back then, but Python is also a good language for this kind of task. Python is suited for this type of task mainly because it has relatively simple syntax and is easy to write. It』s also quick to write something small with it and test it.
What about embedded applications?
I』m not an expert on embedded applications, but I know that Python works with Rasberry Pi. It seems like a popular application among hardware hobbyists.
What about gaming?
You could use the library called PyGame to develop games, but it』s not the most popular gaming engine out there. You could use it to build a hobby project, but I personally wouldn』t choose it if you』re serious about game development.
Rather, I would recommend getting started with Unity with C#, which is one of the most popular gaming engines. It allows you to build a game for many platforms, including Mac, Windows, iOS, and Android.
What about desktop applications?
You could make one with Python using Tkinter, but it doesn』t seem like the most popular choice either.
Instead, it seems like languages like Java, C#, and C++ are more popular for this.
Recently, some companies have started using JavaScript to create Desktop applications, too.
For example, Slack』s desktop app was built with something called Electron. It allows you to build desktop applications with JavaScript.
Personally, if I was building a desktop application, I would go with a JavaScript option. It allows you to reuse some of the code from a web version if you have it.
However, I』m not an expert on desktop applications either, so please let me know in a comment if you disagree or agree with me on this.
Python 3 or Python 2?
I would recommend Python 3 since it』s more modern and it』s a more popular option at this point.
Footnote: A note about back-end code vs front-end code (just in case you are not familiar with the terms):
Let』s say you want to make something like Instagram.
Then, you』d need to create front-end code for each type of device you want to support.
You might use, for example:
- Swift for iOS
- Java for Android
- JavaScript for web browsers
Each set of code will run on each type of device / browser. This will be the set of code that determines what the layout of the app will be like, what the buttons should look like when you click them, etc.
However, you will still need the ability to store users』 info and photos. You will want to store them on your server and not just on your users』 devices so each user』s followers can view his/her photos.
This is where the backend code / server-side code comes in. You』ll need to write some backend code to do things like:
- Keep track of who』s following who
- Compress photos so they don』t take up so much storage space
- Recommend photos and new accounts to each user in the discovery feature
So, this is the difference between backend code and front-end code.
By the way, Python is not the only good choice for writing backend / server-side code. There are many other popular choices, including Node.js, which is based on JavaScript.
Liked this article? Then, you might also like my YouTube channel.
I have a programming education YouTube channel called CS Dojo with 440,000+ subscribers, where I produce more content like this article.
For example, you might like these videos:
What Programming Language Should I Learn First?
Anyway, thanks a lot for reading my article!
每天推薦一個 GitHub 優質開源項目和一篇精選英文科技或編程文章原文,歡迎關注開源日報。交流QQ群:202790710;微博:https://weibo.com/openingsource;電報群 https://t.me/OpeningSourceOrg