每天推荐一个 GitHub 优质开源项目和一篇精选英文科技或编程文章原文,欢迎关注开源日报。交流QQ群:202790710;电报群 https://t.me/OpeningSourceOrg
今日推荐开源项目:《下一代文本编辑器 Xray》
推荐理由:Xray 是 Atom 团队正在新开发的一个基于 Electron 的文本编辑器,目前并没有开发完成,正处于试验阶段。Atom 团队利用自己之前开发 Atom 的经验,并将自己新的想法应用于 Xray,经过快速迭代不断验证,希望能够开发出一个高性能、高可扩展、高兼容、跨平台、适用于任何 Web 应用的文本编辑器。
高性能方面:性能被项目团队设为首要特性,项目对于性能的目标还是比较高的,如下图
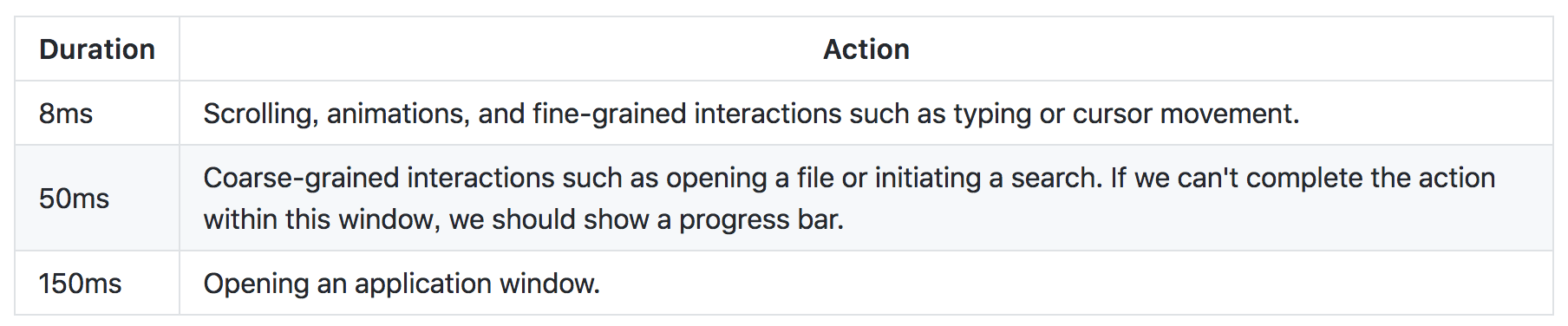
Atom 编辑器推出后性能方面一直被社区和用户诟病,其中在加载大文件的情况下,性能问题尤为明显,因此 Atom 开发团队希望通过 WebGL 将界面这块进行重新实现。但是他们不希望抛弃 Electron,因为他们相信 Electron 还是开发跨平台可扩展界面最优秀的技术平台。
核心逻辑:Xray 相对于 Atom 最大的变动在于核心逻辑层面将由 Rust 来开发。选择 Rust ,其类型系统、并行能力将会使其整体的架构更轻量,并且可维护性更好。但是 Rust 是一门比较偏冷门的语言,并且目前没有太多的项目对其大规模应用,抛弃 node 使用 Rust,不仅仅对团队整体开发是个挑战,对项目之后面向社区提升了门槛。
Atom 和 Xray:就现有的发展来看,Xray 还没有到一个成熟项目阶段,官方也说了各种试验验证还在进行中,并且需要在各种试验之后才能安排出整体项目的开发时间表。因此 Xray 短中期内还没有能力达到 Atom 接班人的水平,但有一点是可以认识到的,在 Xray 的相关技术达到成熟验证后,Atom 团队必定会将大部分开发精力投入到 Xray 当中去。

从 Xray 的整体架构可以看出与 Atom 还是有比较大的差别,Xray 的定位其实不仅仅是一款简单的编辑器而已,Atom 团队希望将 Xray 打造为一款精致的个人编辑器的同时,还能够成为基于 Github 团队协作的一样强大工具,其架构和设想远大。
关于 Xray 的讨论
编辑器这方面从来不缺话题,编辑器是和广大开发同胞息息相关的伴侣。可能从编程开始,它陪伴您的时间比谁都多,因此有时选一款编辑器不亚于选房选车。对于 Atom 团队正在试验的 Xray 项目知乎上有很多有意思的讨论和观点:https://www.zhihu.com/question/268413089 可以关注并仔细思考一番。
以小编的亲身经历来讲,选什么编辑器取决于您选择什么类型的工作什么类型的岗位,小编从早期 turbo pascal, trubo c 到 vim 后,目前 JetBrains 公司的全家桶一直是我的选择。
今日推荐英文原文:《I learned all data structures in a week. This is what it did to my brain》
I learned all data structures in a week. This is what it did to my brain.
Over the last week, I studied seven commonly used data structures in great depth. The impetus for embarking on such a project was a resolution I made at the beginning of the year to train myself to be a better software engineer and write about things I learned in the process.
In the last 3 years since I first studied about them during my undergraduate studies, I felt no glimmer of temptation to study any one of them again; it wasn’t the complex concepts which kept me away, but their lack of usage in my day to day coding. Every data structure I’ve ever used was built into the language. And I’ve forgotten how they worked under the hood.
They were inescapable now. There are seven data structure in the series to be studied.
Let us go back to where it all began. Like every invention has a necessity, and having data structures also had one.
Say you’ve to find a specific book in an unorganized library. You’ve put in numerous hours in shuffling, organizing and searching for the book. The next day the librarian walks in, looks at the mess you’ve created and organizes the library in a different way.
Problem?
Just like you can organize books in a library in 100 different ways, you can structure data in 100 different ways. So, we need to find a way to organize our books(read: data) so that we can find our book(read: data) in a very efficient and fastest way.
Solution :
Luckily for us, some uber smart people have built great structures that have stood the test of time and help us solve our problem. All we need to know how they work and use them. We call them data structures. Accessing, inserting, deleting, finding, and sorting the data are some of the well-known operations that one can perform using data structures.
The first entry in the series ‘Array’ leaves no need to have multiple data structures. And yet there will be so many more. I do not have the energy to describe why one data structure triumph over another one. But, I’ll be honest with you: it does matter knowing multiple data structures.
Still not convinced?
Let’s try to solve few operations with our beloved array. You want to find something in an array, just check every slot. Want to insert anything in middle? You can move every element to make room.
Easy-peasy, right?
The thing is “all of these are slow”. We want to find/sort/insert data efficiently and in the fastest possible way. An algorithm may want to perform these operations a million of times. If you can’t do them efficiently, many other algorithms are inefficient. As it turns out, you can do lots of things faster if you arrange the data differently.
You may think, “Ah, but what if they ask me trivia questions about which data structure is most important or rank them”
At which point I must answer: At any rate, should that happen, just offer them this — the ranking of the data structures will be at least partially tied to problem context. And never ever forget to analyze time and space performance of the operations.
But if you want a ranking of learning different data structures, below is the list from most tolerable to “oh dear god”
- Array
- Stacks
- Queues
- Linked List
- Hash Tables
- Trees
- Graphs
You will need to keep the graph and trees somewhere near the end, for, I must confess: it is huge and deals with zillions of concepts and different algorithms.
Maps or arrays are easy. You’ll have a difficult time finding a real-world application that doesn’t use them. They are ubiquitous.
As I worked my way through other structures, I realized one does not simply eat the chips from the Pringles tube, you pop them. The last chip to go in the tube is the first one to go in my stomach(LIFO). The pearl necklace you gifted your Valentine is nothing but a circular linked list with each pearl containing a bit of data. You just follow the string to the next pearl of data, and eventually, you end up at the beginning again.
Our Brain somehow makes the leap from being the most important organ to one of the world’s best example of a linked list. Consider the thinking process when you placed your car keys somewhere and couldn’t remember.Our brain follows association and tries to link one memory with another and so on and we finally recall the lost memory.
We are connected on Medium. Thank you, Graphs. When a data structure called trees goes against nature’s tradition of having roots at the bottom, we accept it handily. Such is the magic of data structures. There is something ineffable about them — perhaps all our software are destined for greatness. We just haven’t picked the right data structure.
Here, in the midst of theoretical concepts is one of the most nuanced and beautiful real-time examples of the stacks and queues data structure I’ve seen in real life.
Browser back/forward button and browsing history
As we navigate from one web page to another, those pages are placed on a stack. The current page that we are viewing is on the top and the first page we looked at is at the base. If we click on the Back button, we begin to move in reverse order through the pages. A queue is used for Browsing history. New pages are added to history. Old pages removed such as in 30 days
Now pause for a moment and imagine how many times we, as both a user and developer, use stacks and queues. That is amazing, right?
But, my happiness was short-lived. As I progressed with the series, I realized we have a new data structure based on a doubly-linked list that handles browser back and forward functionality more efficiently in O(1) time.
That is the problem with the data structures. I am touched and impressed by a use case, and then everyone starts talking about why one should be preferred over other based on time complexities and I feel my brain cells atrophying.
In the end, I am left not knowing what to do. I can’t look at the things the same way ever again. Maps are graphs. Trees look upside down. I pushed my article in Codeburst’s queue to be published. I wish they introduced something like prime/priority writers, which might help me jump the queue. These data structures look absolutely asinine yet I cannot stop talking/thinking about them. Please help.
每天推荐一个 GitHub 优质开源项目和一篇精选英文科技或编程文章原文,欢迎关注开源日报。交流QQ群:202790710;电报群 https://t.me/OpeningSourceOrg