今日推薦開源項目:《ChinesePinyin-CodeCompletionHelper》
今日推薦英文原文:《How AI Will Make Nuclear Energy More Affordable》
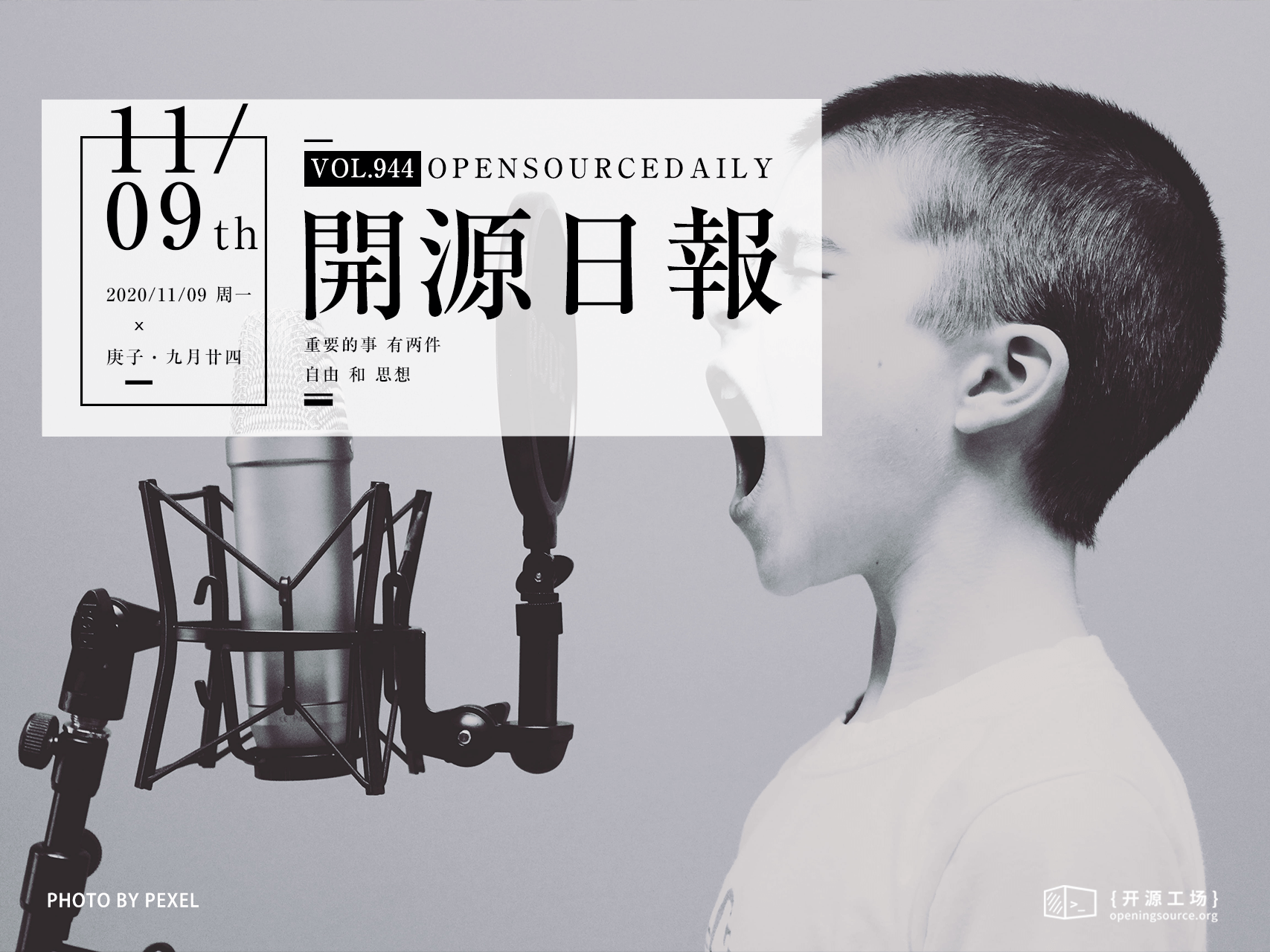
今日推薦開源項目:《ChinesePinyin-CodeCompletionHelper》傳送門:項目鏈接
推薦理由:中文編程又來了,這次支持的是 JetBrains 全家桶,有拼音輸入補全,多音字無差別補全等功能,起名困難症患者的好幫手。
今日推薦英文原文:《How AI Will Make Nuclear Energy More Affordable》作者:Priya Aggarwal
原文鏈接:https://medium.com/generation-atomic/how-ai-can-make-nuclear-energy-cheaper-d2d2c1e4ab3
推薦理由:和其他傳統工業不同,核工業鑒於它的特殊性,其機械化,智能化程度相對要低很多。不過更加先進的人工智慧或許可以解決之前機器解決不了的問題。
How AI Will Make Nuclear Energy More Affordable
AI can eliminate many manual and tedious tasks and bring down design, operation, and waste management costs.
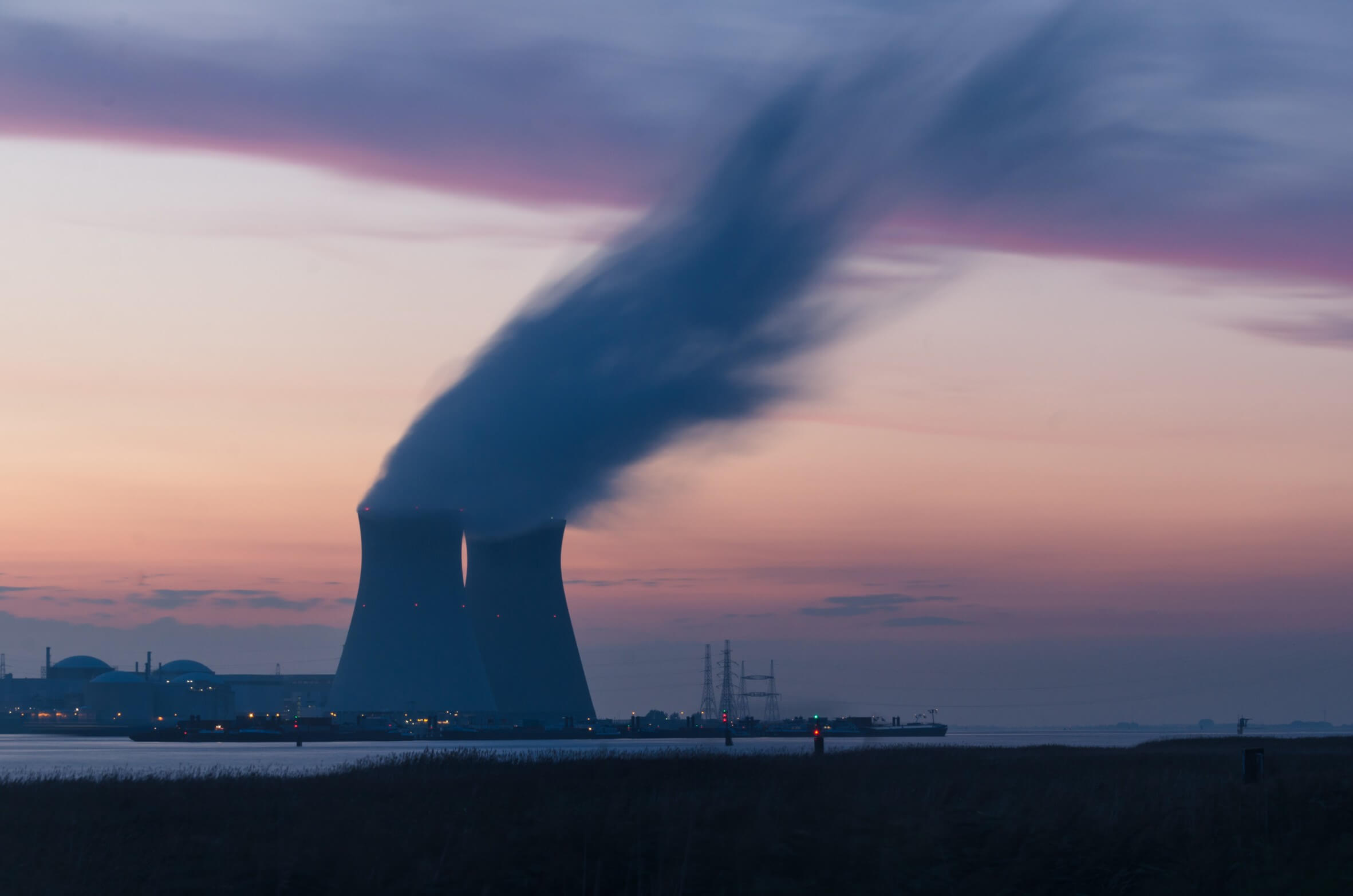
(Photo by Frédéric Paulussen on Unsplash)Nuclear power is one of the cheapest forms of generating carbon-free energy but is instead known for being the opposite. While constructing a nuclear plant is expensive and recent projects in the US and EU have suffered from overruns, operating it is cheaper than many other energy sources. It also turns out that the reason for expensive construction is not entirely technical and often has political factors outside the control of the maker. So how can these costs be reduced?
A nuclear plant's costs are made up from capital and operation costs. Capital costs include site preparation, engineering, manufacturing, construction, commissioning, and financing. Operating costs include fuel costs, maintenance, decommissioning, and waste disposal.
Capital costs can make up to 60% of the expense, if not more. Recent years have seen the launch of many startups who claim smaller, more modular designs will bring down the costs. The engineering, manufacturing, and construction expenses could come down a lot with pre-approved designs and economies of scale in their manufacturing.
What will also help is lowering design, maintenance, and decommissioning costs by automating many manual and tedious tasks using Artificial Intelligence. These tasks mostly need a highly skilled workforce and special processes when dealing with radioactive materials, whether for research, maintenance, or disposal.
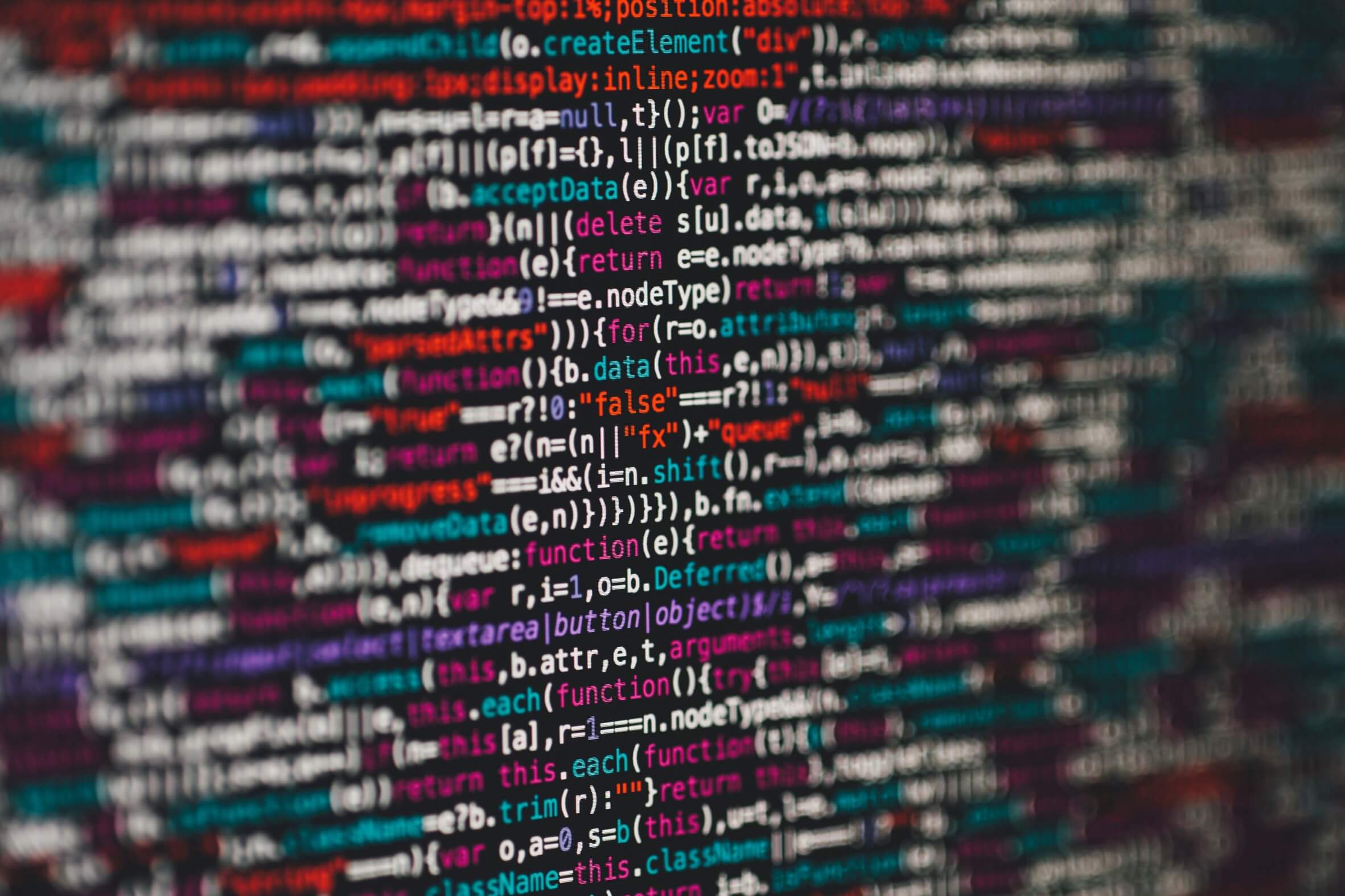
(Photo by Markus Spiske on Unsplash)
Artificial Intelligence for Nuclear Energy
The field of AI is vast and researchers are trying to make nuclear technology more efficient by predicting accidents, material interactions, and radioactive leaks.It』s not just researchers who are interested; some of the biggest nuclear plant designers like GE are also exploring machine learning techniques for predictive maintenance. They are coming up with 'digital twins' that can might help to bring down O&M costs by up to 10 times.
Here are some other examples of the many applications being studied.
Deep Learning-Based Crack Detection
Due to high temperatures and radiation that can damage a material, the reactor needs to be constantly inspected. This is a tedious yet highly skilled task that requires humans to watch hours of video footage. Reactor parts could also be underwater, which makes analyzing videos all the more difficult and prone to errors since cracks can be tiny and hazy. The study to make visual detection simpler with different algorithms claims to have noticed a 98.3% accuracy.Predicting void swelling in irradiated materials
Neutrons are produced as part of the nuclear reaction. Over time, constant bombardment by neutrons can degrade the reactor vessel and impact its performance. There is a limit to how much a material can take before needing replacement. Much effort has been spent on predicting these changes and to develop more radiation-resistant materials to extend a reactor's life, but this remains a challenge. Using machine learning, researchers are trying to predict the neutron 'dose' a metal can take before swelling starts and understand key factors that contribute to it.Robots for nuclear waste
It is surprising how un-roboticised nuclear industry is, given its unconventional nature. But things may be changing. With more sophisticated robots trained to do specialized tasks like identifying, handling, and decommissioning waste, robots can carry out time-consuming tasks in zones where it is better to limit human access as radiation levels are high. Robots are also being trained for imaging radioactivity that can help in identifying residual contamination in facilities on walls, floors, etc.Modeling Nuclear Processes
Argonne National Lab is trying to use machine learning to simulate the underlying mechanics that govern a nuclear reactor and then use it to improve safety and operations when designing a new reactor. For example, many thermal-hydraulic processes can be difficult to predict because of heavy computation requirements, but machine learning can help identify key factors influencing heat flow with faster running models.Since the advent of nuclear energy, it has been one of the safest and cleanest forms of power generation, albeit not the cheapest to set up. But with more standardization and AI, costs are expected to go down as economies of scale, predictive maintenance and safer designs will allow designers to optimize how reactors are operated and maintained to bring down costs. This will allow them to compete with the now rock-bottom price of shale gas.
下載開源日報APP:https://openingsource.org/2579/
加入我們:https://openingsource.org/about/join/
關注我們:https://openingsource.org/about/love/